Mount Sinai medical researchers claim ChatGPT is ready to practice medicine
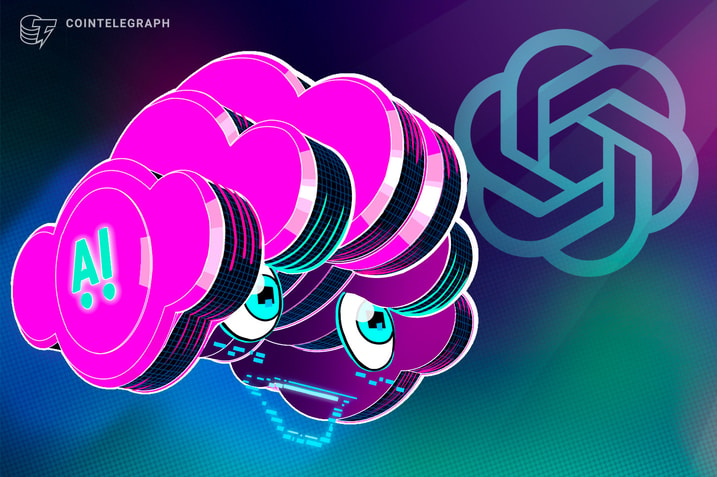
While the researchers claim this important first step could change the medical field, their paper leaves a lot undiscussed.
A team of medical researchers from the Icahn School of Medicine at Mount Sinai recently conducted a study on artificial intelligence (AI) chatbots wherein they determined that “generative large language models are autonomous practitioners of evidence-based medicine.”
The experiment
According to preprint research published on arXiv, the Mount Sinai team tested various off-the-shelf consumer-facing large language models (LLMs), including both ChatGPT 3.5 and 4 and Gemini Pro, as well as open-source models LLaMA v2 and Mixtral-8x7B.
The models were given prompts engineered with information such as “you are a medical professor” and then asked to follow evidence-based medical (EBM) protocols to suggest the proper course of treatment for a series of test cases.
Once given a case, models were tasked with suggesting the next action, such as ordering tests or starting a treatment protocol. They were then given the results of the action and prompted to integrate this new information and suggest the next action, and so on.
According to the team, ChatGPT 4 was the most successful, reaching an accuracy of 74% over all cases and outperforming the next-best model (ChatGPT 3.5) by a margin of approximately 10%.
This performance led the team to the conclusion that such models can practice medicine. Per the paper:
“LLMs can be made to function as autonomous practitioners of evidence-based medicine. Their ability to utilize tooling can be harnessed to interact with the infrastructure of a real-world healthcare system and perform the tasks of patient management in a guideline directed manner.”
Autonomous medicine
EBM uses the lessons learned from previous cases to dictate the trajectory of treatment for similar cases.
While EBM works somewhat like a flowchart in this way, the number of complications, permutations and overall decisions can make the process unwieldy.
As the researchers put it:
“Clinicians often face the challenge of information overload with the sheer number of possible interactions and treatment paths exceeding what they can feasibly manage or keep track of.”
The team’s paper indicates that LLMs can mitigate this overload by performing tasks usually handled by human medical experts, such as “ordering and interpreting investigations, or issuing alarms,” while humans focus on physical care.
“LLMs are versatile tools capable of understanding clinical context and generating possible downstream actions,” write the researchers.
Current limitations
The researchers’ findings may be somewhat biased by their professed perception of the capabilities of modern LLMs.
At one point, the team writes, “LLMs are profound tools that bring us closer to the promise of Artificial General Intelligence.” They also make the following claim twice in the document: ”We demonstrate that the capacity of LLMs to reason is a profound ability that can have implications far beyond treating such models as databases that can be queried using natural language.”
However, there’s no general consensus among computer scientists that LLMs, including the foundational models underpinning ChatGPT, have any capacity to reason.
Can language models learn to reason by end-to-end training? We show that near-perfect test accuracy is deceiving: instead, they tend to learn statistical features inherent to reasoning problems. See more in https://t.co/2F1s1cB9TE @LiLiunian @TaoMeng10 @kaiwei_chang @guyvdb
— Honghua Zhang (@HonghuaZhang2) May 24, 2022
Furthermore, there’s even less consensus among scientists and AI experts as to whether artificial general intelligence is possible or achievable within a meaningful time frame.
The paper doesn’t define artificial general intelligence or expand on its authors’ declaration that LLMs can reason. It also doesn’t mention the ethical considerations involving the insertion of an unpredictable automated system into existing clinical workflows.
LLMs such as ChatGPT generate new text every time they’re queried. An LLM might perform as expected during testing iterations, but in a clinical setting there’s no method by which it can be constrained from occasionally fabricating nonsense — a phenomenon referred to as “hallucinating.”
Related: OpenAI faces fresh copyright lawsuit a week after NYT suit
The researchers claim hallucinations were minimal during their testing. However, there’s no mention of mitigation techniques at scale.
Despite the researchers’ benchmarks, it remains unclear what benefits a general chatbot such as ChatGPT would have in a clinical EBM environment over the status quo or a bespoke medical LLM trained on a corpus of curated, relevant data.
… [Trackback]
[…] Info to that Topic: x.superex.com/news/ai/1841/ […]
… [Trackback]
[…] Find More on that Topic: x.superex.com/news/ai/1841/ […]
… [Trackback]
[…] There you will find 69742 more Information to that Topic: x.superex.com/news/ai/1841/ […]