The topic of MCP is trending across the internet, the best bridge for AI to break free from data silos
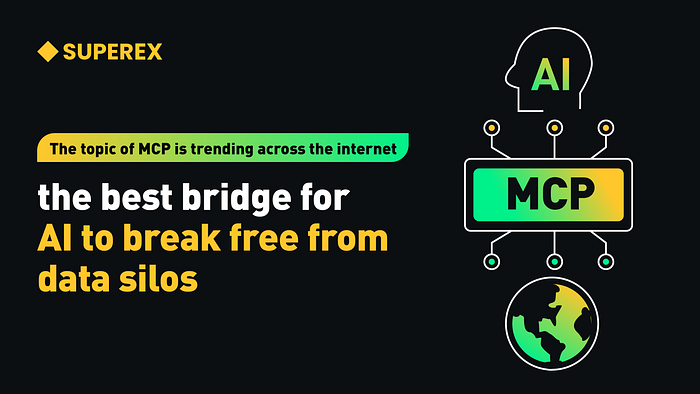
#MCP #AI #AIAgent
Recently, some market analysts have suggested that the continued downtrend of Web3 AI Agent tokens such as #ai16z and $arc is caused by the recent surge in popularity of the MCP protocol. Unbelievable! Is there a connection between the two? Of course, there is. Once you understand what the MCP protocol is, you’ll realize that its emergence has directly reshaped the AI narrative and stripped AI Agents of their former “halo.”
MCP, short for Model Context Protocol, is an open, standardized protocol designed to seamlessly connect various AI LLMs/Agents to different data sources and tools. It functions like a universal plug-and-play USB interface, replacing the previous approach of requiring end-to-end “specific” encapsulation. With MCP, AI systems can interact with external resources more flexibly, breaking past data silos and enabling real-time, dynamic data access and collaborative operations.
Simply put, AI applications originally operated within distinct data silos. For Agents/LLMs to communicate and share resources, they had to develop their own respective API interfaces, making the process not only complex but also lacking in bidirectional interaction capabilities. Typically, access to models and permissions was relatively limited. This limitation was precisely the fundamental reason for the growth of the AI Agent market — these agents served as “data bridges,” filling the gaps where direct model-to-model connections were difficult to establish.
- Click to register SuperEx
- Click to download the SuperEx APP
- Click to enter SuperEx CMC
- Click to enter SuperEx DAO Academy — Space

And the emergence of MCP provides a unified framework that allows AI applications to break free from past data silos, enabling “dynamic” access to external data and tools. This significantly reduces development complexity and improves integration efficiency, particularly in areas such as automated task execution, real-time data queries, and cross-platform collaboration.
With the MCP protocol, AI models are no longer restricted by the limitations of a single data source or tool but can interact with external systems in a standardized manner. By defining a unified standard for data access and operations, MCP enables different types of AI models to seamlessly share data and resources.This very aspect has started to erode the technical barriers and commercial value that AI Agents previously built around data isolation.
The Impact of the MCP Protocol on AI Agents
Before the introduction of MCP, the market demand for AI Agents primarily stemmed from the need to bridge AI models with external tools. Due to the closed and independent nature of various models, many business scenarios required dedicated Agents to facilitate data transmission and task coordination between models. However, this approach was not only costly to develop but also lacked flexibility in real-world applications — any change in data sources or tools necessitated adjustments to interfaces and calling methods.
The emergence of the MCP protocol has broken this deadlock by providing a unified interface standard, enabling AI applications to achieve fast and dynamic data access and operations across various scenarios. From a technical perspective, the open nature of MCP reduces reliance on intermediary Agents, allowing developers to focus on optimizing models and expanding business use cases rather than dealing with the complexities of underlying data integration.
The most direct impact of this shift is the contraction of the AI Agent market. The traditional advantage of AI Agents in handling integration complexity has been completely replaced by MCP’s standardization. In the Web3 landscape, projects that positioned AI Agents as their core value proposition initially gained market share by capitalizing on the challenges posed by “data silos.” However, as MCP gains widespread adoption, these projects’ competitive advantages are rapidly diminishing, leading to the sustained decline in their market valuations.
Not only that, but MCP’s open-source nature has also attracted a growing number of developers and organizations. The active participation and collaborative efforts of the developer community have gradually enhanced the MCP ecosystem, making the integration of various data tools and resources increasingly diverse. A wide range of third-party tools, data providers, and DApp developers are now adopting the MCP protocol to leverage its advantages in data fluidity and openness.
From a macro perspective, the introduction of MCP as a standardized protocol has also brought a new turning point to the AI narrative in the Web3 space. Traditional AI Agents, which once functioned as an intermediary layer, are gradually losing their technical barriers. Meanwhile, MCP, serving as an open bridge for data and tools, is emerging as the new dominant force in the ecosystem.
From a technical standpoint, the MCP protocol not only provides a standardized interface for data access but also comes with built-in functional modules, such as dynamic data parsing, multi-model parallel computing, and cross-platform task coordination. These features significantly enhance the efficiency of AI applications and simplify complex task processing.
For example, in multi-model parallel computing, MCP enables developers to quickly integrate multiple LLMs, allowing for more complex task scheduling. It even facilitates multi-language, multi-domain data synchronization and real-time feedback, unlocking new possibilities for AI applications across different industries.
In-Depth Analysis of the Relationship Between the MCP Protocol and the AI Agent Market
The core value of the MCP protocol lies in its standardization and decoupling capabilities. Traditional AI Agent development requires customized interface development for each target data source or tool, which involves several pain points:
- High repetitive development costs: For example, if a medical AI Agent needs to connect with five different hospitals’ electronic medical record (EMR) systems, it must develop five independent API adapters.
- Poor scalability: If a new pharmaceutical database is introduced, the underlying code must be modified to integrate it.
- Fragmented permission management: Different data sources use their own authentication mechanisms (such as OAuth, API Keys, etc.), making security policies difficult to unify.
How MCP Solves These Issues Through a Three-Layer Architecture
MCP addresses these challenges through a three-layer architectural design:
- Protocol Layer: Defines standardized data request formats (such as JSON Schema) and interaction protocols (REST/gRPC).
- Adaptation Layer: Provides a universal data converter, supporting automatic translation between different query languages (e.g., SQL to GraphQL).
- Governance Layer: Integrates fine-grained access control, supporting dynamic role binding and data usage proof mechanisms.
This design significantly improves development efficiency. Test data shows that the average time to integrate a new data source has been reduced from two weeks (traditional method) to just four hours, leading to an 87% reduction in human resource costs.
This is precisely the direct reason behind the decline in AI Agent project valuations. Imagine this: what once required a professional team several months to develop — a complex data bridge — can now be achieved instantly through MCP protocol configuration.
Written at the End
In conclusion, the emergence of the MCP protocol has not only redefined the collaborative model of AI but also disrupted the existing market structure, placing AI Agents under unprecedented pressure for transformation. And the market is the most sensitive, has quickly reflected this pressure in the declining valuations of AI Agent-related projects. It is evident that the technological innovations brought by MCP pose both challenges and demands for adaptation in the AI Agent space.
Projects that rely on “data silos” as their selling point will face the risk of being phased out if they fail to quickly adapt to MCP’s widespread adoption. On the other hand, projects and developers that successfully integrate into the MCP ecosystem will have a significant advantage in this AI-driven transformation.
At the same time, MCP will continue to enhance its protocol standards, supporting more advanced features such as multi-model parallel computing, real-time task scheduling, and multi-domain collaborative processing. This will drive AI applications toward deeper levels of intelligent collaboration and data sharing.
At the end of the article, let’s leave a topic for discussion: “As an open protocol, does MCP still have the possibility of collaboration, supplementation, or coexistence? For example, can AI Agents still execute more complex business logic or scenario-based operations based on the MCP protocol, rather than merely serving as data bridges?”
Everyone is welcome to share your thoughts!

Responses