What are deepfakes, and how to spot fake audio and video?
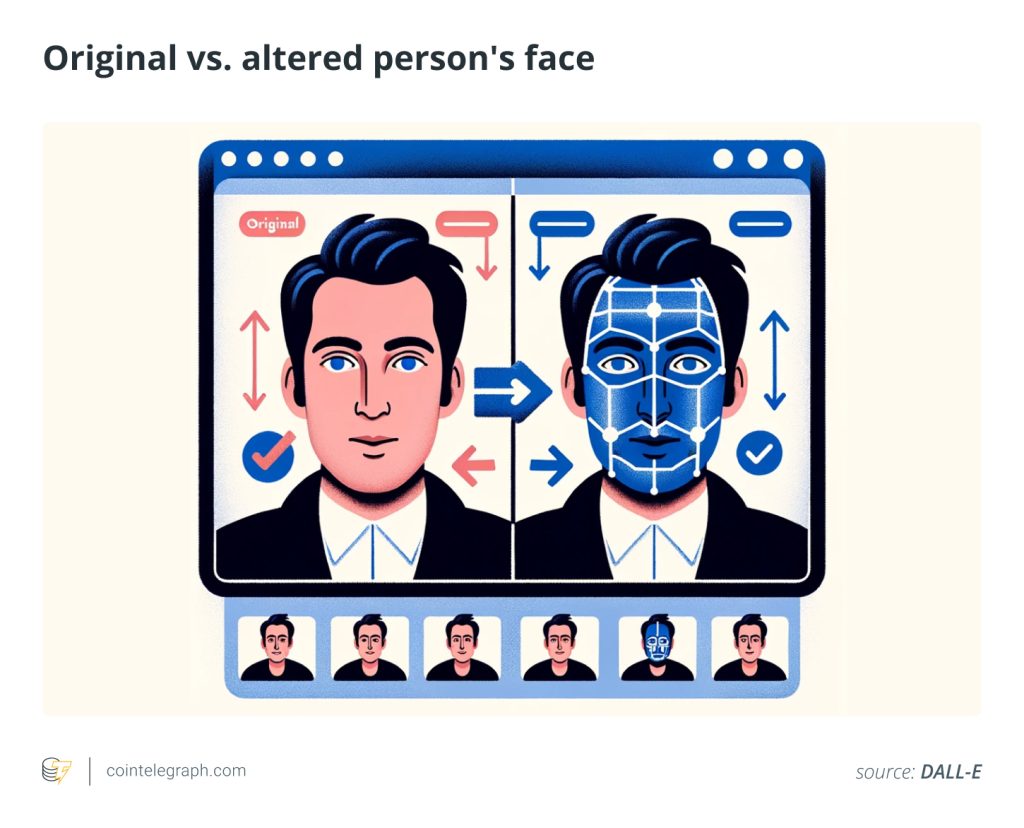
In an era where digital content forms the cornerstone of information dissemination and interpersonal communication, the veracity of the media being consumed has never been more critical. However, with the advent of artificial intelligence (AI) and machine learning (ML), the digital realm is becoming a fertile ground for misinformation and deception, primarily through the burgeoning phenomenon known as deepfakes.
What are deepfakes?
Deepfakes are hyper-realistic synthetic media created by leveraging sophisticated machine learning algorithms, predominantly generative adversarial networks (GANs). This technology can fabricate videos and audio recordings that are incredibly convincing, often portraying real individuals saying or doing things they never did. The term “deepfake” is a portmanteau of “deep learning” and “fake,” which succinctly encapsulates the essence of this technology.
The emergence of deepfakes, facilitated by advancing technology, opens a perilous chapter in digital misinformation, challenging the authenticity of digital media by spreading false narratives, damaging reputations and threatening national security. The malicious use of deepfakes has permeated political, corporate and personal domains, accentuating the need for effective detection methods to differentiate real from fake content.
This article endeavors to demystify the technical aspects of deepfake creation, identify synthetic media signs, and navigate through deepfake detection technologies, aiming to uphold truth in the digital arena amid the growing menace of deepfake technology.
How do deepfakes work?
At the heart of deepfake technology are GANs, a class of artificial neural networks. In a GAN setup, two neural networks, namely the generator and the discriminator, are pitted against each other in a cat-and-mouse game.
The generator strives to create realistic synthetic media, while the discriminator distinguishes between real and generated content. Through continuous training, the generator becomes proficient in crafting increasingly convincing fake media, often to a degree where the synthetic content becomes indistinguishable from real content to the human eye and ear.
The following steps will explain how deepfakes are created:
Data collection
Accumulating a substantial data set comprising images, videos or audio recordings of the target individual.
Preprocessing
Preparing the collected data by aligning images, normalizing audio levels and other data cleaning tasks to ensure consistency and quality.
Training
Feeding the preprocessed data into the GAN enables the networks to learn and improve over numerous iterations.
Generation
Producing synthetic media once the GAN is adequately trained using new input data or combining elements from the training data to fabricate convincing fake content.
Ethical concerns of deepfakes
Deepfake technology, while having benign uses like entertainment and education, poses significant ethical concerns due to its potential for malicious applications such as misinformation and fraud.
The core issue with deepfakes lies in their ability to convincingly depict individuals in false scenarios, leading to damaged reputations, social unrest and even national security threats. The ease of creating and spreading deepfakes amplifies the challenge of upholding digital media integrity. Understanding the technology behind deepfakes and developing effective detection methods is essential to counter these risks.
Privacy violations
The creation of deepfakes often involves using personal images or videos without consent, leading to privacy violations. This is particularly concerning in cases where deepfakes are used to create fake explicit content or other malicious purposes.
Legal and ethical accountability
The ease of creating and disseminating deepfakes raises questions about legal and ethical accountability. Determining who is responsible for the harm caused by deepfakes is challenging, as is establishing laws and regulations that can keep pace with rapidly evolving technology.
Erosion of public trust
Deepfakes contribute to the erosion of public trust in media and institutions. As it becomes increasingly difficult to distinguish between real and fake content, the public’s trust in information sources can significantly diminish, impacting societal cohesion and informed decision-making.
Exploitation and harassment
Deepfakes can be used for exploitation and harassment, particularly targeting women and minorities. The creation of non-consensual fake explicit content or the use of deepfakes for bullying and harassment are grave concerns that highlight the need for ethical guidelines and protective measures.
How to spot fake video and audio
Detecting deepfake videos is a multifaceted challenge, necessitating a blend of technical acumen and sophisticated analytical tools. The following subsections will explain how to spot a fake video:
Ways to spot a fake video
Audio synchronization
A hallmark of deepfake videos often lies in the subtle inconsistencies between audio and visual elements. One common discrepancy is the lack of synchronization between the audio and the mouth movements of the individuals in the video. Analyzing the coherence between audio cues and corresponding visual actions can unveil anomalies indicative of a deepfake.
Eye reflections
The eyes, often termed windows to the soul, can also act as windows to the truth in digital media. Inconsistencies in the reflections observed in a subject’s eyes, or unnatural eye movements, can betray the artificial nature of a video. A meticulous analysis of eye reflections, gaze directions and other ocular indicators can shed light on the authenticity of the video content.
Unnatural movement
The fluidity and naturalness of movement are intricate to replicate artificially. Deepfake videos may exhibit unnatural facial expressions, lack of blinking or inconsistent head movements, which are red flags for synthetic content. Analyzing the movement dynamics and comparing them with natural movement patterns can be instrumental in identifying deepfake videos.
Detection algorithms
The frontier of deepfake detection has seen the advent of specialized algorithms designed to identify the subtle anomalies often present in synthetic media. Techniques like convolutional long short-term memory (LSTM), eye blink detection and grayscale histograms have been explored for their efficacy in deepfake detection.
These algorithms delve into the minute details of video content, analyzing frame-by-frame discrepancies, temporal inconsistencies and other technical indicators to ascertain the authenticity of the media.
Deepfake detection challenge
Community-driven initiatives like the deepfake detection challenge provide a platform for researchers and practitioners to advance detection technologies. These initiatives foster a collaborative environment for developing and evaluating novel detection algorithms, propelling the field toward more robust and reliable deepfake detection solutions.
Ways to spot a fake audio
The realm of audio deepfakes, though less visually apparent, holds equally menacing potential for misinformation and deception. The ability to synthesize realistic audio can result in fabricated voice recordings, posing significant risks in a world heavily reliant on auditory communication.
Fluid dynamics
The texture of natural human speech possesses a fluid dynamism that is intricate to replicate synthetically. Researchers have ventured into analyzing minute inconsistencies in audio deepfakes using principles of fluid dynamics. By meticulously examining the acoustic waves and their propagation, anomalies that deviate from natural speech patterns can be identified, aiding in the detection of synthetic audio.
Frame-by-frame analysis
Deepfake audio detection often necessitates a granular analysis of the audio frames, which are small segments or slices of the audio track. Employing frame-by-frame analysis facilitates the identification of artificially generated or modified audio content. The authenticity of audio content can be evaluated by scrutinizing each frame for anomalies like inconsistent pitch, tempo or other acoustic properties.
Neural models
The realm of deepfake audio detection has witnessed the development of sophisticated neural models designed to discern genuine audio from synthetic counterparts. Models like Resemble Detect employ state-of-the-art neural networks to analyze audio frame-by-frame in real time, identifying and flagging any artificially generated or modified audio content. Such models delve into the nuances of acoustic properties, offering a promising avenue for real-time detection of deepfake audio.
CNN techniques
Convolutional Neural Networks (CNNs) have emerged as a potent tool in the arsenal against deepfake audio. CNNs for fake audio detection utilize spectrogram representations to analyze audio segments, aiming to discern anomalies, inconsistencies, or artifacts indicating manipulation or synthesis. The utilization of CNNs in deepfake audio detection exemplifies the blend of deep learning and acoustic analysis, paving the way for robust detection mechanisms.
Importance of public awareness in detecting AI-generated deepfakes
Education and awareness form the first line of defense against deepfake deception. By informing the public about the signs of deepfake media and promoting the use of reliable verification tools, a collective vigilance against misinformation can be nurtured. Educational campaigns, workshops and online resources are instrumental in spreading awareness about deepfake technology and its potential dangers.
Promoting the use of reliable verification tools empowers individuals and organizations to ascertain the authenticity of digital content. Tools like DeepFake-o-meter, although no longer publicly available, exemplify the kind of resources that can provide a level of assurance against deepfake deception. Encouraging the development and accessibility of such tools is a significant stride toward fostering a more secure digital ecosystem.
The future of deepfake technology
The rapid evolution of deepfake technology foretells a concerning trajectory in digital misinformation. As distinguishing between real and synthetic media becomes more complex, the urgency for developing adept detection mechanisms intensifies.
The malicious use of deepfakes for political, corporate or personal deceit highlights an imminent necessity for collective efforts in combating this menace. The ongoing battle against deepfake technology requires a united front from the tech community, policymakers and the public to ensure a trustworthy digital ecosystem.
As the venture ahead unfolds, fostering public awareness, advancing detection technologies, and promoting a culture of digital discernment are pivotal to mitigating the threats posed by deepfakes and preserving authenticity in the digital domain.
Written by Tayyub Yaqoob
… [Trackback]
[…] Find More on on that Topic: x.superex.com/academys/beginner/1945/ […]
… [Trackback]
[…] Find More on that Topic: x.superex.com/academys/beginner/1945/ […]
… [Trackback]
[…] Here you will find 88999 additional Information on that Topic: x.superex.com/academys/beginner/1945/ […]
… [Trackback]
[…] Read More here on that Topic: x.superex.com/academys/beginner/1945/ […]
… [Trackback]
[…] Read More Information here on that Topic: x.superex.com/academys/beginner/1945/ […]
… [Trackback]
[…] Find More to that Topic: x.superex.com/academys/beginner/1945/ […]
… [Trackback]
[…] Here you will find 53091 additional Information on that Topic: x.superex.com/academys/beginner/1945/ […]
… [Trackback]
[…] Find More here on that Topic: x.superex.com/academys/beginner/1945/ […]
… [Trackback]
[…] Read More to that Topic: x.superex.com/academys/beginner/1945/ […]
… [Trackback]
[…] Info on that Topic: x.superex.com/academys/beginner/1945/ […]
… [Trackback]
[…] Read More to that Topic: x.superex.com/academys/beginner/1945/ […]
… [Trackback]
[…] There you can find 35342 more Info on that Topic: x.superex.com/academys/beginner/1945/ […]
… [Trackback]
[…] There you will find 47844 additional Info on that Topic: x.superex.com/academys/beginner/1945/ […]
… [Trackback]
[…] Read More on on that Topic: x.superex.com/academys/beginner/1945/ […]