The ABCD of AI: Automation, big data, computer vision and deep learning
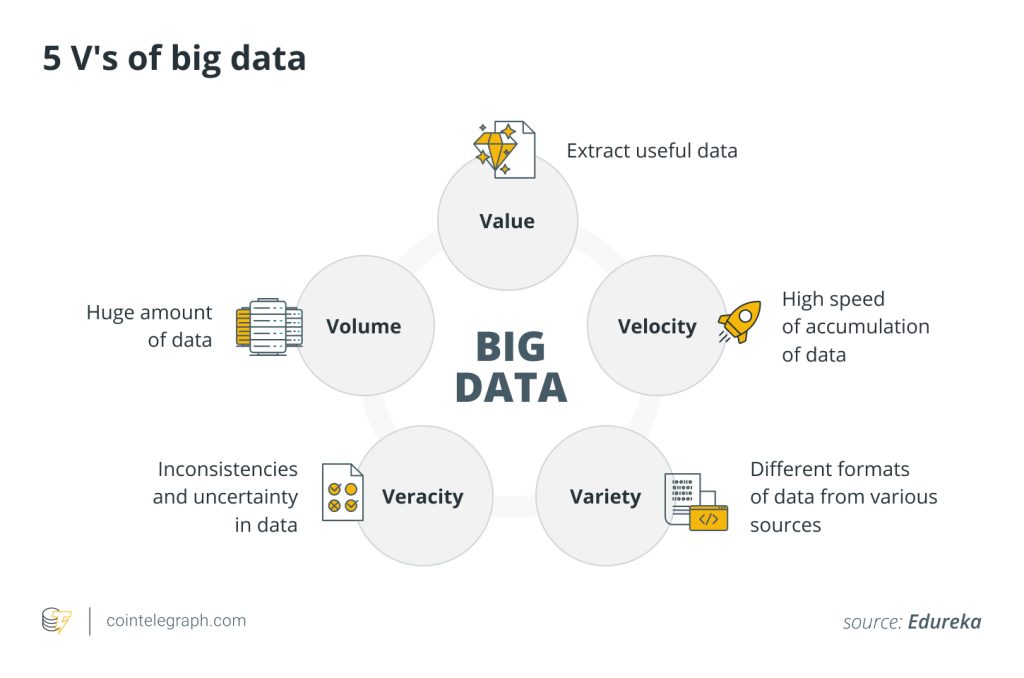
Introduction to the ABCD framework
Artificial intelligence (AI) is a revolution that is changing how individuals live and work; it’s not just a technological development. There are fundamental building blocks for AI that outline its application and potential. Automation, big data, computer vision and deep learning are the four pillars that make up what is referred to as the “ABCD of AI,” the main technologies advancing this revolution.
Automation
In the context of AI, automation refers to a machine’s or system’s capacity to carry out activities autonomously. This is made possible through the use of algorithms and software that can perform particular tasks — from easy to difficult — without requiring human input.
Automation helps transform industries by replacing tiresome, repetitive labor with smart, self-executing procedures. Automation streamlines operations, improves productivity, and optimizes workflows by using algorithms and intelligent systems.
Automation is being embraced by businesses and industries all around the world to increase productivity, lower error rates, and devote human resources to more innovative and important duties.
Big data
Big data serves as the engine for AI systems. Due to the exponential rise of digital information, businesses make use of enormous databases to uncover insightful information. This vast amount of data is processed by AI systems at scales and rates that are unimaginable to humans.
Businesses can make data-driven decisions, improve strategies, and forecast market trends by looking for patterns, trends and correlations. This promotes innovation and competitive advantage.
Computer vision
Computer vision is a branch of artificial intelligence that gives computers the ability to decipher and comprehend visual data. It enables computers to examine image or video data and draw conclusions from it.
From facial recognition systems and driverless vehicles to medical imaging and augmented reality, this technology has a wide range of applications. AI systems interact with the world in a way that mimics human vision when visual intelligence is incorporated, improving security, practicality and user experiences.
Deep learning
Neural networks that learn from enormous data sets are a key component of deep learning, which is motivated by the structure and operation of the human brain. These networks are able to identify complex patterns, enabling AI systems to carry out activities that were previously thought to be unique to human intellect.
Deep learning can be used for speech recognition, language translation and sophisticated decision-making. The development of advanced AI applications is fueled by their capacity to continuously learn from and improve upon data, transforming industries such as healthcare, finance and entertainment.
How ABCD components of AI work together in artificial intelligence systems
Let’s understand how automation, big data, computer vision and deep learning are related and can work together in the context of cryptocurrencies and blockchain technology, using fraud detection and prevention and supply chain and provenance tracking as examples.
Fraud detection and prevention
Data collection and analysis (big data)
Massive volumes of data, such as transaction histories, timestamps and user behavior, are generated by cryptocurrency transactions. Big data technologies, such as Apache Hadoop and Spark, gather and examine this data to look for trends and anomalies that can point to fraudulent activity, including unlawful transactions or trading irregularities.
Hadoop and Spark are both big data processing frameworks, but Spark is quicker because of in-memory computing, making it suitable for real-time data analysis. Batch processing is appropriate for Hadoop because it is based on disk storage. Spark is a popular option for contemporary, data-intensive applications due to its speed and adaptability.
Pattern recognition (deep learning)
Recurrent neural networks or convolutional neural networks (CNNs), in particular, are powerful deep learning algorithms that can scan complicated transaction data sequences and spot small patterns that might point to fraud. Deep learning algorithms, for instance, can recognize regular transaction patterns and indicate abnormalities to help spot possibly fraudulent transactions.
Authentication and identity verification (computer vision)
In the course of user onboarding procedures on cryptocurrency platforms, computer vision technologies may be employed for facial recognition and document verification. Computer vision algorithms can check the identity of users, preventing identity theft and providing secure access to cryptocurrency accounts by examining facial features and ID papers.
Automated responses (automation)
Automated systems have the ability to react instantly to trends in fraud. For instance, an automated response can freeze the account and initiate multifactor authentication procedures to verify the user’s identification if a deep learning model identifies a suspicious transaction and the user fails facial recognition verification.
Supply chain and provenance tracking
Supply chain data (big data)
Big data technologies gather and store data about the supply chain, such as details on products, shipping history and manufacturing procedures. Throughout the supply chain, this data promotes transparency and traceability.
Product authentication (computer vision and deep learning)
Images of products can be examined by computer vision systems to ensure their validity and quality. For instance, a computer vision system can verify a product’s legitimacy by comparing images of the product received in a shipment with reference images kept on the blockchain.
Moreover, deep learning techniques, such as CNNs, can help improve the precision of product verification using image recognition. These algorithms ensure efficient and adaptable verification processes by being able to identify abnormalities, evaluate product quality, and make decisions about product authenticity in real-time.
Immutable record keeping (blockchain)
With the use of blockchain technology, every step of the supply chain process is transparently and irrevocably recorded. The blockchain records every transaction, from production to delivery, ensuring the legitimacy and integrity of the product’s journey.
Smart contracts for transaction (automation)
The automation of transactions and agreements between parties participating in the supply chain is made possible by smart contracts on the blockchain. For instance, a smart contract can immediately start the payment process when a package is delivered and validated by a computer vision system, ensuring fast and automated transactions.
The ethical challenges associated with AI
The rapid advancement of AI technology raises profound ethical concerns. Since AI systems frequently rely on enormous volumes of personal data, privacy is one of the main concerns. This raises issues with consent and data security.
Bias and discrimination are major issues since AI systems may pick up prejudices from the data they are trained on, which could result in unjust treatment of some groups. The complexity of AI models makes it difficult to understand how decisions are made, which hinders accountability in the event of mistakes or biases, complicating transparency and accountability.
Another concern is the disruption of employment, as job losses brought on by automation may have an effect on livelihoods and economies. AI in warfare also raises ethical problems about the morality of utilizing technology for harmful ends, as autonomous systems may do.
To ensure the responsible development and application of AI technology, these issues call for strong legislation, moral standards and ongoing communication between technologists, legislators and society.
Future trends in artificial intelligence
The trajectory of artificial intelligence is being shaped by various key trends that hope for revolutionary advancements. For instance, AI is set to become more pervasive and find use in a variety of industries, including healthcare, banking and transportation. Deep learning, in particular, will advance machine learning models’ capacity to perform challenging tasks like image recognition and natural language processing.
Furthermore, it is anticipated that the fusion of blockchain and AI will completely transform data security and transparency. The integrity of AI algorithms and the data they process can be ensured due to the blockchain’s decentralized and tamper-proof design. AI-powered smart contracts will enable automated, safe and transparent transactions, revolutionizing a variety of industries from financial services to supply chain management.
Explainable AI (XAI) is another critical trend. There is an increasing demand for transparency and interpretability as AI systems get more complex. The main goals of XAI are to increase trust and accountability by making AI algorithms intelligible and making sure that judgments made by AI models can be justified.
It is also interesting that edge AI is growing. Edge computing is used in Internet-of-Things devices and autonomous systems because it enables data processing to take place closer to the source of the data, reducing latency and increasing efficiency.
Furthermore, ethical AI development and responsible AI deployment will be at the forefront. The creation and application of AI systems will be influenced by ethical factors, such as bias reduction, fairness and data protection, to ensure that society as a whole benefits. The future of AI seems not only intelligent but also ethically sound and socially beneficial as these tendencies unfold.
Written by Tayyub Yaqoob
… [Trackback]
[…] Find More Info here to that Topic: x.superex.com/academys/beginner/2222/ […]